How Makimoto’s Wave Explains the Tsunami of Specialized AI Processors Headed for Market
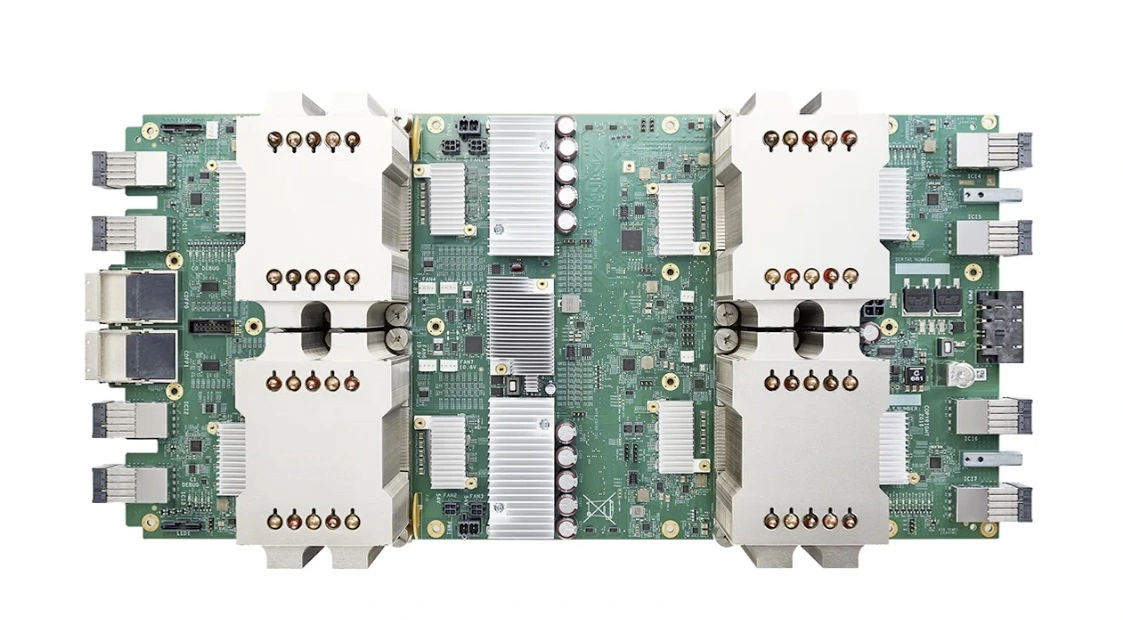
There are certain terms of art in semiconductor technology that have become common touchstones. Moore’s law, Dennard scaling, and concepts like the “memory wall” refer to long-established trends in technology that often resurface across many different areas of expertise. In that vein, there’s a concept we’re going to discuss today that some of you may be familiar with, but that we haven’t previously covered here at ET: Makimoto’s wave. While it doesn’t date back quite as far as Gordon Moore’s seminal paper, Makimoto’s argument has direct bearing on the booming market for AI and machine learning devices.
First presented in 1991, Makimoto’s wave is a way of describing the fact that the semiconductor market often swings between specialization and standardization. These cycles have often occurred in roughly 10-year intervals, though there’s been disagreement in the larger space about whether or not the 1997-2007 and 2007-2017 cycles were strong enough to qualify.
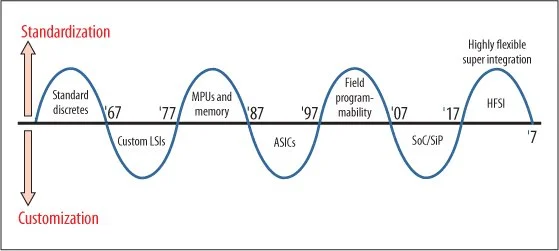
The theory isn’t contested for earlier cycles, however. From 1957-1967, standardized discrete components dominated the market, followed by custom large-scale integration chips, which gave way to the first standardized microprocessor and memory technologies.
It’s not clear that Makimoto’s classic wave, as shown above, cleanly aligns with the current push into AI and ML. It predicts that the market should be moving towards standardization starting in 2017, when in reality we’re seeing a tremendous push from a wide range of companies to build their own custom accelerator architectures for specialized AI and ML workloads. With everyone from Fujitsu and Google to Nvidia and AMD throwing a proverbial hat into the ring, the pendulum seems to be arcing farther towards customization, not already in the middle of swinging back towards standardization.
But it’s not unusual for a generally accepted theory that explains some aspect of semiconductor progress to fail to map perfectly to real life. Moore’s law, in its original incarnation, predicted the doubling of transistor counts every single year. In 1975, Gordon Moore revised his prediction to every two years. The actual rate at which transistor counts have doubled in shipping products has always varied somewhat depending on foundry node transition difficulties, market conditions, and the success or failure of CPU design teams. Even Moore’s law scaling has slowed in recent years, though density improvements have not yet stopped. After Dennard scaling quit in 2004, density scaling became the only metric continuing to follow anything like its old historical path.
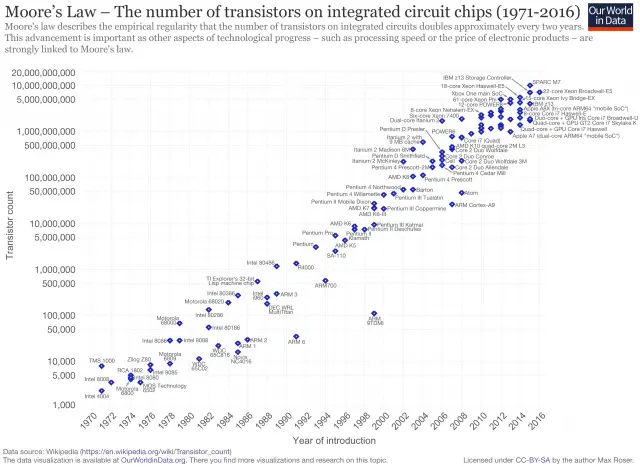
And given how drastically general purpose CPU scaling has changed between earlier eras and the present day, we have to allow for the fact that the pendulum may not swing exactly the same way that it used to. The video below, narrated by Makimoto, isn’t new — it was published in 2013 — but it offers a further explanation of the concept to anyone interested.
A new article at SemiEngineering details the rush of firms working on specialized accelerator architectures and why the field is red-hot. Faced with the lack of progress in general-purpose compute, companies have turned their attention to accelerators, in the hopes of finding workloads and cores that map well to one another. Thus, it might seem as if the pendulum is swinging permanently away from general-purpose compute.
But this is effectively impossible in the long term. While there’s nothing stopping a firm from developing a specialized architecture to process a well-known workload, not every workload can be described in such a manner. As Chris Jones, vice president of marketing at Codasip, told SemiEngineering: “There always will be instances where the software that will be run on a given chip is largely unknown, and if the software load is indeterminate, all the chip designer can do is provide a robust general compute platform where performance is purely a function of core frequency and memory latency.”
In other words, you can’t simply build an array of hardware accelerators to cover every workload. General-purpose compute remains critical to the process. Custom implementations of work also become standardized over time as companies zero in on optimal implementations for handling certain kinds of work.
There’s some significant overlap between the behavior Makimoto’s wave describes and the looming accelerator wall we discussed earlier this week. The accelerator-wall paper demonstrates that we can’t depend on accelerator solutions to provide infinite performance improvements absent the ability to improve underlying aspects of transistor performance via Moore’s law. Makimoto’s wave describes the broad industry trend to oscillate between the two. The recent flood of venture-capital money into the AI and machine learning markets has led to a definite hype-cycle around these capabilities. AI and machine learning may indeed revolutionize computing in years to come, but the new trend towards using accelerators for these workloads should be understood within the context of the limits of that approach.
Continue reading
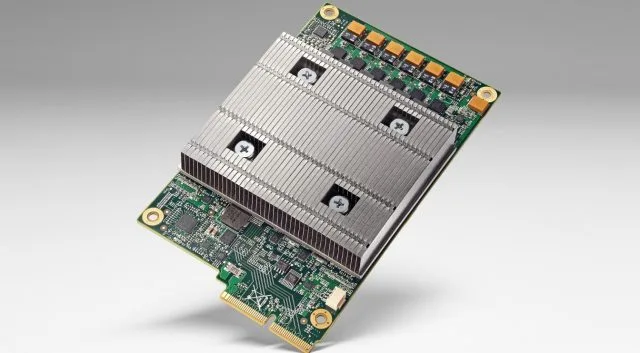
Specialized Chips Won’t Save Us From Impending ‘Accelerator Wall’
Hardware accelerators won't solve the fundamental performance scaling issues facing us when Moore's law runs out of gas.